Highlights:
- Generative models, like large language models (LLMs) and foundation models (FMs), have the power to uncover trillions in economic value by notably boosting productivity.
- AI operationalization is necessary to unveil its full potential and foster business value.
The generative AI market is experiencing explosive growth, projected to expand at a staggering 24.40% annually, according to Statista’s 2023 report.
AI is transforming industries with unmatched efficiency and innovation, but its intricacies present major challenges. Hence, organizations must ensure AI systems are ethical, effective, compliant, and transparent.
Additionally, to fully uncover AI while reducing risks, a robust AI governance framework is required. By imposing clear-cut guidelines, tracking systems, and accountability measures, organizations can save their reputation, develop trust, and help drive speedier and more sustainable AI adoption.
Cracking the AI Code: Barriers to Expanding AI’s Reach
AI’s supremacy is constantly enhancing as leaders across various industries adopt the technology. Nevertheless, many enterprises struggle with significant challenges that come their way while implementing AI. It includes:
-
Operationalizing AI with confidence:
Regardless of the availability of various AI governance tools, models are often created with insufficient clarity, monitoring, or documentation.
As a result, the lack of comprehensive, automated AI lifecycle tracking affects transparency and scalability and makes explainable results hard to attain.
-
Concerns with “Black Box” models:
AI models, once created and deployed, frequently lags clarity in decision-making procedures, making it confusing to get the rationale behind decisions—even for the data scientists who created them.
Consequently, it can lead to inefficiencies such as scope drift, delays or failures in production, conflicting quality, and neglected risks.
-
Managing risk and reputation is hard:
Managing risk and reputation is hard, with headlines about biased or unexplainable AI models. Incorrect assumptions can affect your brand in a bad manner.
Thus, utilizing explainable processes ensures transparency and helps prevent bias in complex fields like transaction reviews, patient diagnoses, and loan applications.
Hence, developing fair, transparent, and inclusive AI systems is significant for controlling privacy, security, and customer trust.
-
AI regulations are changing:
Compliance with regional, local, and national laws is utterly important, as non-compliance can lead to hefty fines reaching tens of millions, like those explained in the EU AI Act.
Thus, proper model documentation is needed, especially as new rules will require comprehensive metadata and lineage records. Ensure your enterprise stays ahead by taking this crucial step.
AI Models with Modern Capabilities
AI models can vary in quality, but they all require in-depth management and monitoring. While many businesses still use traditional machine learning, there’s growing interest in generative AI.
Machine learning models are developed using algorithms trained on data. These models learn to highlight patterns and make predictions without human advice. Different algorithms suit different tasks, and models enhance with more data.
Generative models
Generative models, such as large language models (LLMs) and foundation models (FMs), have the immense power to unveil trillions in economic value by boosting productivity.
These models are versatile, scalable, customizable, and cost-effective, processing large amounts of data while continuously learning. Off-the-shelf generative applications need minimal expertise and can automate repetitive tasks.
Conventionally used in statistics to verify numerical data, generative models have recently extended through deep learning to generate images, music, speech, video, text, and code.
They are applicable in various sectors, like marketing, customer service, retail, and education.
While these models have increased AI’s importance for business leaders, their modern capabilities initiate new complexities and risks for enterprises and society.
AI for Governance: All Models Need Governance
To employ AI effectively, you need a robust cross-functional team. AI is a strategic need for many leaders, and the list of stakeholders involved seems to keep growing.
Some are new to the AI lifecycle, while others have new reasons to engage in AI efforts. It’s necessary to meet their expectations without pressuring your data scientists, who are already pressed for time and can’t control all the approvals and information requests.
Start by aligning your stakeholders. Secure buy-in from key parties and include them in ideation, aligning on outcomes, and employing responsible AI practices.
Make sure that the right metrics, KPIs, and objectives are defined in line with your enterprise’s business controls and regulations. Moreover, monitor the metrics identified for your AI models.
Organizational Approaches to AI Governance
Effective AI model governance needs cooperation among legal experts, technologists, business leaders, and ethicists. As AI becomes more integrated into society, effective governance is increasingly necessary to ensure its positive impact.
Effective AI governance goes ahead simply by meeting regulations. It needs a full-fledged system to oversee and manage AI applications.
Large organizations need a broad approach to control their AI systems. Let’s explore a potential roadmap for achieving this.
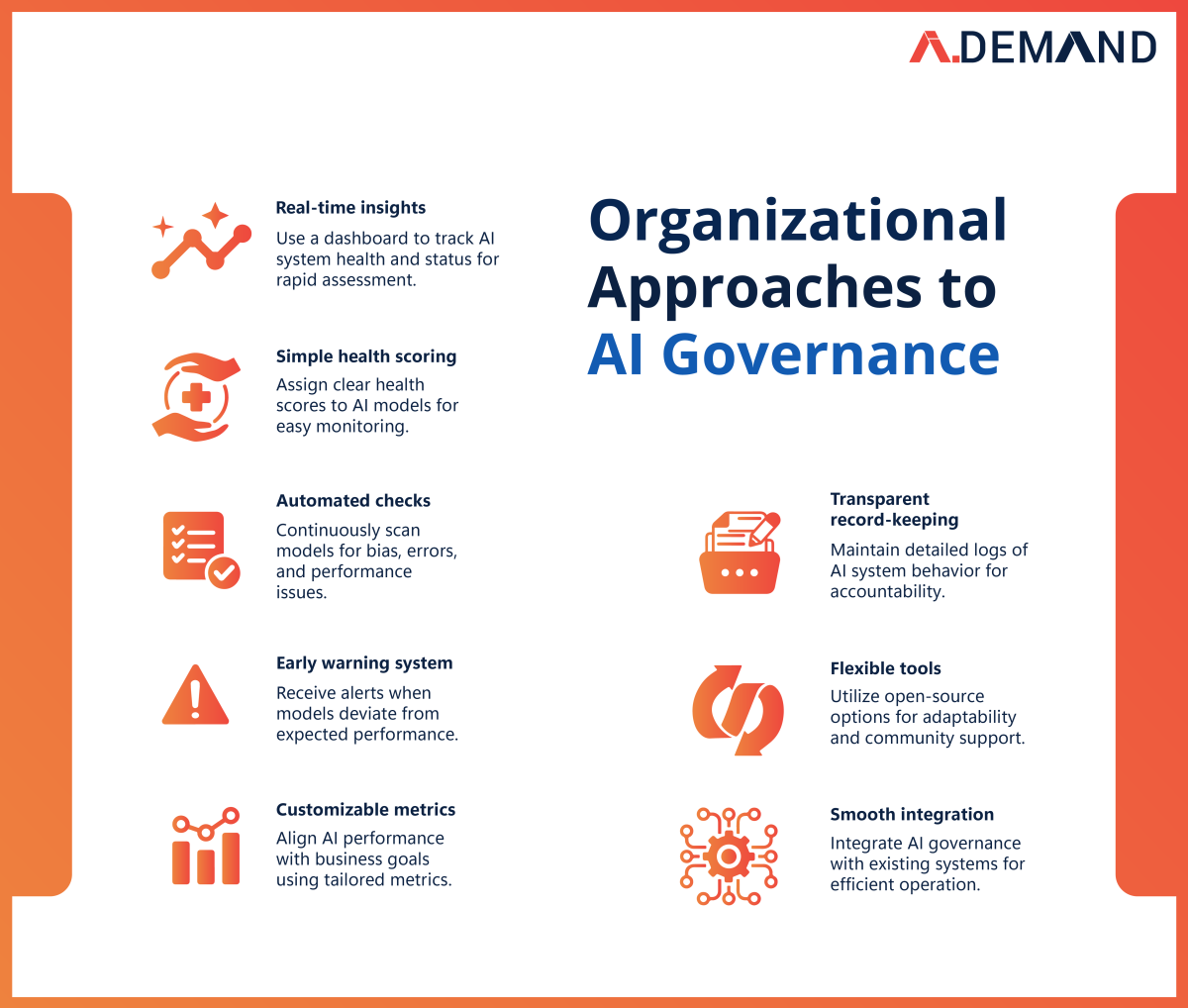
Concluding Remarks
Operationalizing AI is important for realizing its fullest potential and fostering business value. Businesses concentrate on developing advanced AI models as well as integrating them into their core processes.
This needs a modern approach, from setting clear business goals to deploying and optimizing models constantly.
Nevertheless, various challenges remain, like the need for strong AI governance frameworks, the intricacy of controlling “black box” models, and the changing regulatory landscape.
To get through this, businesses need to inculcate broad AI governance practices that guarantee transparency, scalability, and accountability throughout the AI lifecycle.
Finally, a holistic pathway to AI governance needs collaboration among technologists, legal experts, and business leaders. It ensures that AI systems are not only effective but also ethical and compliant.
Enhance your expertise by accessing a range of valuable AI-related whitepapers in our resource center.